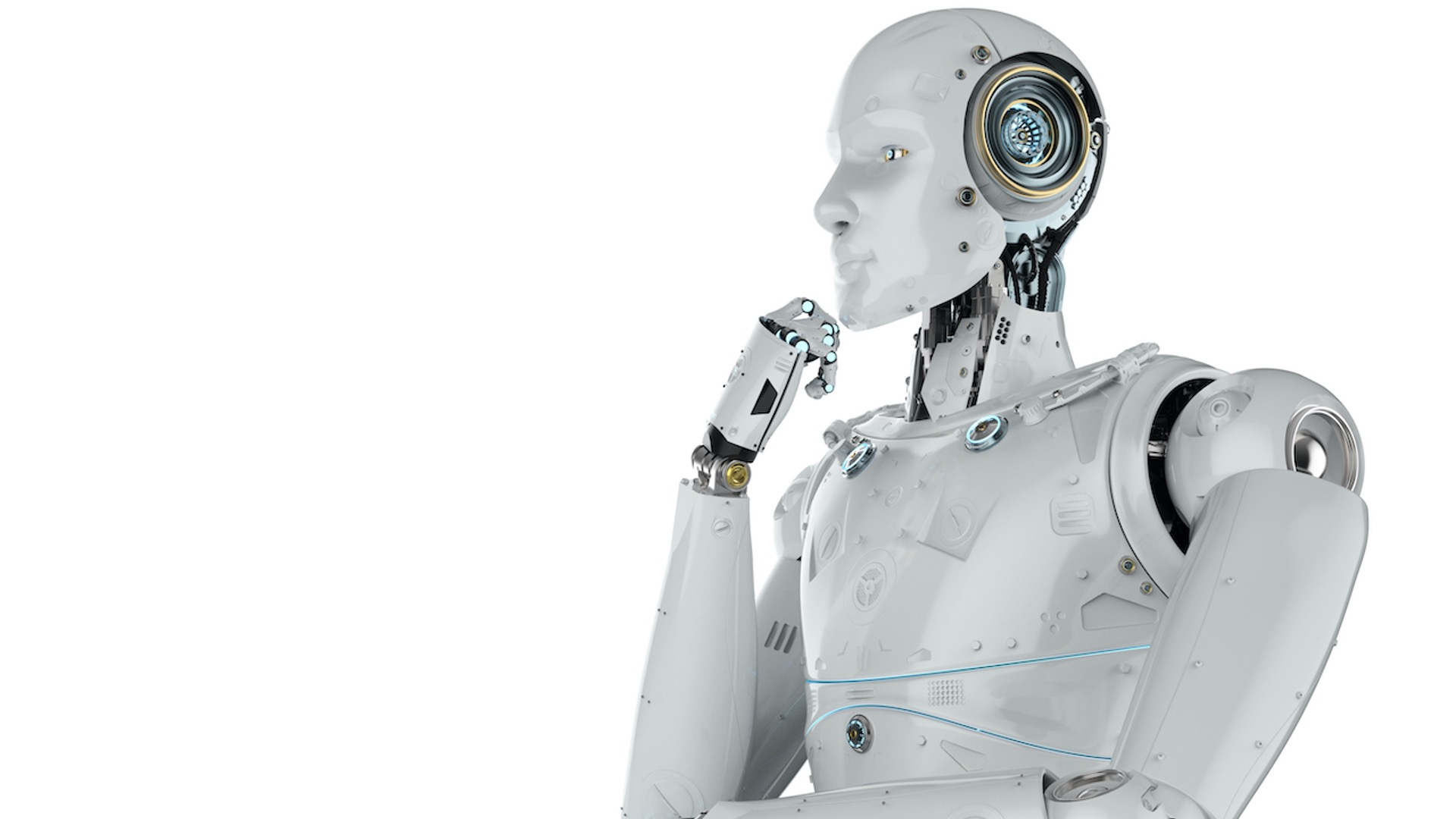
The demand to automate, robotize and make the utmost use of machines for stereotypical and repetitive tasks is rising enormously in the present day. These tasks often put excessive pressure on people due to the need for maximum concentration or physical exertion. How can the implementation of artificial intelligence (AI) help?
In the following paragraphs, you will learn about the five basic steps that need to be taken to implement AI into quality control.
Step 1: When is the use of AI in quality control appropriate?
During quality control, we search for the most common product defects, which have been identified and named. However, not all processes are suitable for automated quality control.
- Looking for random defects, which are not known beforehand, can be very difficult and even impossible in some cases.
- A machine has great endurance and ability to focus, but it isn’t able to deal with dynamic, random situations.
Another aspect of deploying artificial intelligence is the planned quantity and speed required for quality control. AI can help effectively if you need to inspect many parts in a short time and deal immediately with a variety of defects. Artificial intelligence can also control different products running on the same production line simultaneously. However, if you only need to check one product per hour and this check takes half a minute, it is probably pointless to involve AI in quality control.
Step 2: Correct definition of defects
In the previous step, you learned when the use of artificial intelligence is appropriate. We will now take a look at the process during which artificial intelligence gradually learns to process the required tasks.
When a person gets a new job or task, they first need to information or instructions about what to do. Likewise, AI needs to know the definition of the defects it should detect in the production process. They generally work with past experience and the definition of known defects or flaws which have occurred previously. We then define limit values for these defects, which determine whether the defect is still acceptable or requires review, or whether the product must be discarded.
However, because humans evaluate product quality subjectively, these thresholds are not always exact. When using artificial intelligence, this limit is defined using examples or a testing sample. The testing sample consists of a set of images which depict the incidence of the given detect that you wish to detect in various situations. The number of images needed for the testing sample depends on the variability of the defect and complexity of its detection. The definition of defects is largely based on historical experience, which is why at this point, you should work in close cooperation with operators on the production line or quality managers, who should have already determined this definition or be capable of creating the test sample.
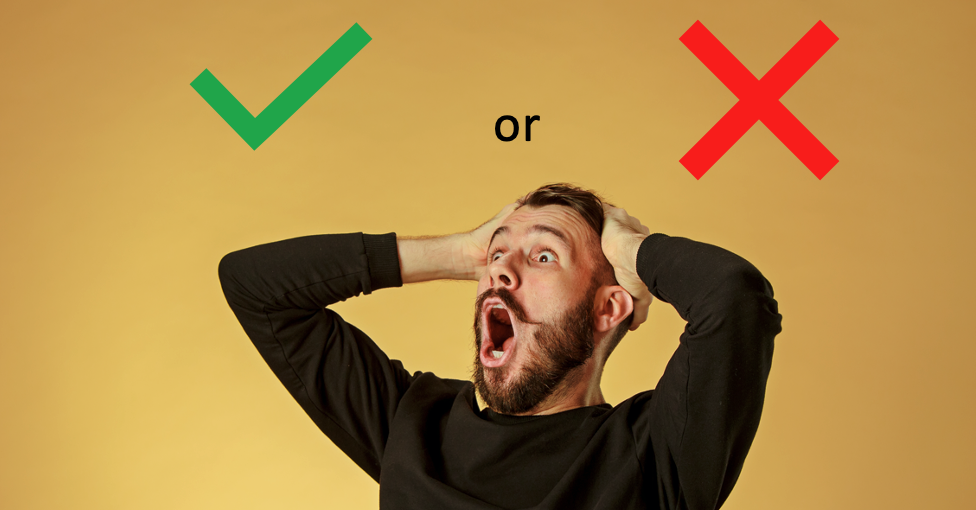
End of part one. If you are interested in how artificial intelligence learns from datasets and how it creates them, click on the next part of our post.