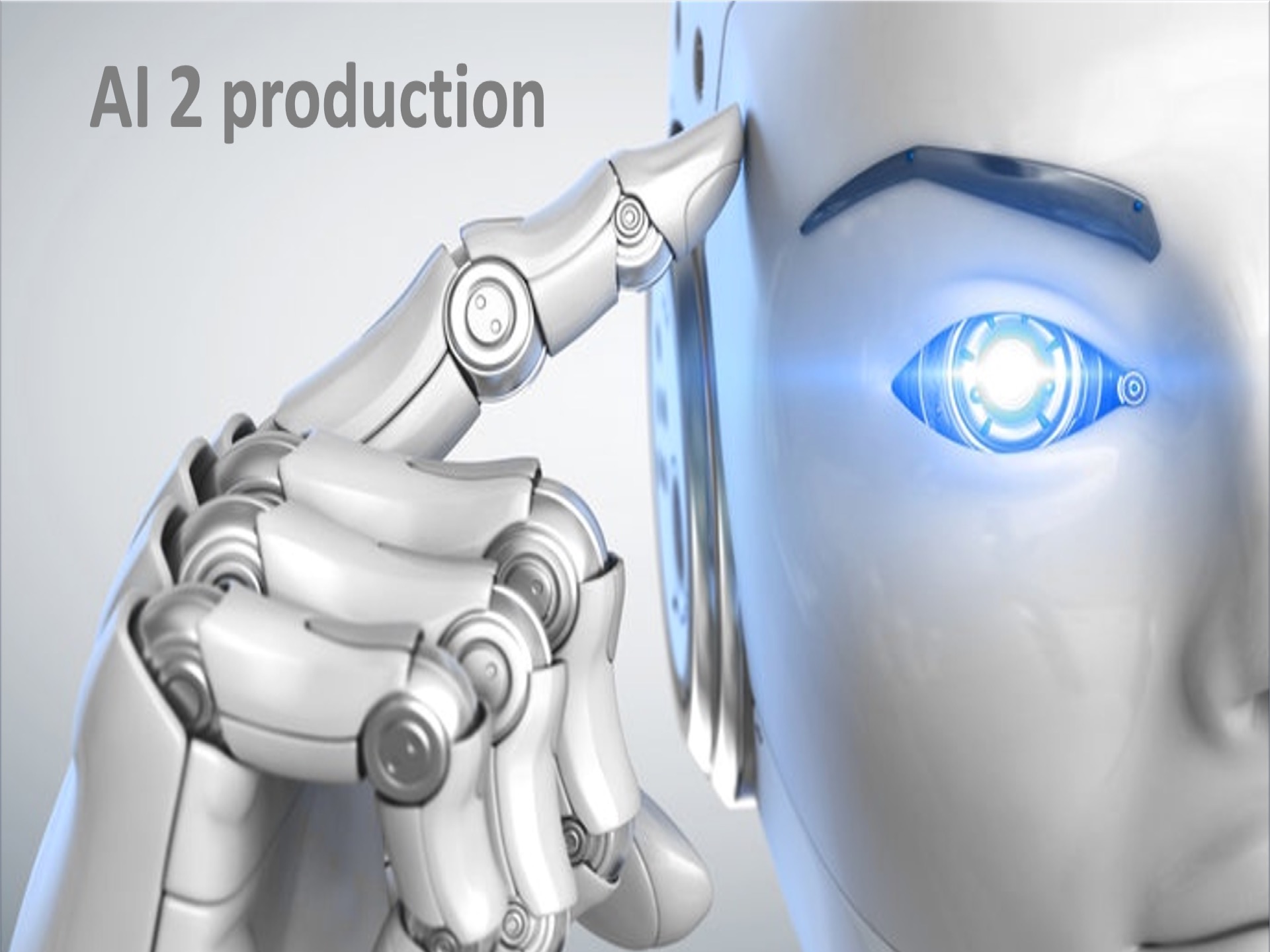
In the second part of our post, you learned about the process of teaching artificial intelligence how to gather experience. We will now move on to production.
Step 4: Integrating artificial intelligence into the production process
As soon as you have enough quality input data and have managed to select and teach the neural network (NN) models to correctly detect what is required, the only thing left is to integrate the entire system into the production process. However, there are at least three more things that need to be configured:
- Input trigger: Firstly, you need to define how to inform the cameras that they are supposed to take pictures. Generally, an analogue or digital trigger from the PLC (production line) is used to send the signal (“Hello, a product is in position!”). The input trigger can also consist of a sensor or other mechanical device. The trigger then serves to inform the cameras, lights or any other devices that action is required and to take pictures.
The input trigger is usually linked to the entire workflow of actions performed by artificial intelligence: AI determines what type of product is on the production line, based on which it evaluates what needs to be checked. Depending on the definition of control, it informs the cameras to take specific pictures, and sends information to the collaborating robot to travel the trajectory and take the required pictures using the camera and defined program. Afterwards, the captured images are passed to the neural network for detection. Depending on the detection results and defined conditions, the product is categorized and its quality defined.
- Analysis output (output trigger): The detection results provide information as to whether or not the product is OK. It is essential for production to define where this information should be sent. It might be to the PLC, to let the production line know whether the product can travel on to dispatching, be rerouted to repair, or be discarded. Line operators also need to receive this information so as to be informed about product quality in real time and be able to take corrective action. The information is also sent to the ERP or MES system, or to the quality portal for subsequent LEAN analysis and production optimization.
- Safety i.e. mechanical assurance of health protection. Occupational safety when implementing equipment into operation is of equal importance. Be it a static inspection station or inspection robots, it is essential to ensure that the premises are secured against the possibility of an accident. These measures can include laser barriers, mechanical safeguards, automatic shutdown of the line in case of human intrusion, etc.
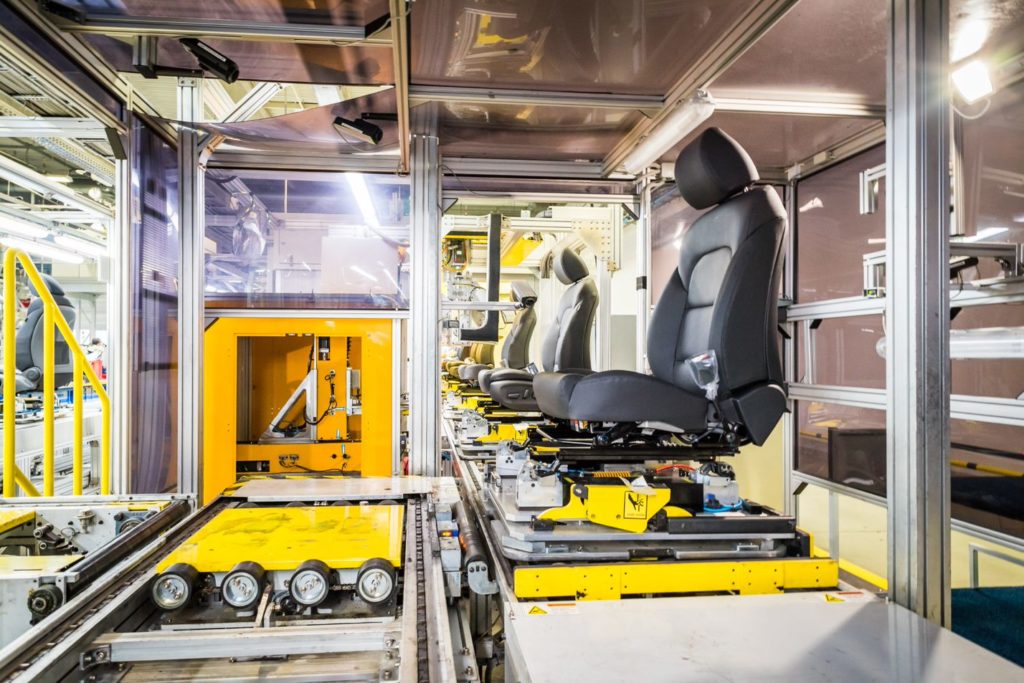
Step 5: Optimization period
The last step in the entire process is optimization. Even after the control station has been implemented and integrated into the production line and artificial intelligence knows the required steps, including testing on an adequate number of samples, you should still stipulate an optimization period in the process. This can last a few days or weeks. During this period, you should still have an operator on the line who verifies the AI results. Based on an analysis of these results, the neural network (NN) is retrained to achieve better results. This process can significantly improve error detection, commonly from 99 % to 99.9 %. You will also ascertain whether there are any accidental, unexpected phenomena in the production, such as a decrease of lighting at night, a change of line speed in crisis situations, etc.
The aforementioned steps are an essential basis for implementing AI. You are likely to encounter more in every specific case. However, it is advisable to be aware of them from the very beginning, to be able plan and underpin these activities.
If you have any questions concerning this topic, please contact us. We will be happy to help you at 24 Vision.